As artificial intelligence (AI) applications make their way into the contact center and unified communication sectors, IT professionals need to pay close attention to the assumptions behind the algorithms as well as the data used for machine learning (ML) purposes.
For example, an AI application designed to support a contact center agent might deliver misleading prompts if it couldn’t understand a caller’s speech. Transcriptions of online conversations are another instance where AI can make mistakes. After all, everyone who has dictated a text or email on a smartphone knows that the speech-to-text application can come up with glaring errors, and should be reviewed before pressing the send button.
A different type of problem can occur when training an AI algorithm using historical data. Your organization might have decades of big data available on issues like seasonal call center volumes or social media click-throughs. But in today’s highly volatile environment, consumer behaviors can change quickly, as shown by the COVID-19 pandemic.
That means it may be more important to focus on small data for machine learning purposes rather that mining big data – one of the key insights from Gartner’s recent EME Virtual Data & Analytics Summit.
“Disruptions such as the COVID-19 pandemic is causing historical data that reflects past conditions to quickly become obsolete, which is breaking many production AI and machine learning models,” said Jim Hare, distinguished research vice president at Gartner. “In addition, decision making by humans and AI has become more complex and demanding, and overly reliant on data hungry deep learning approaches.”
Instead, IT leaders need to turn to “small data” and “wide data” analytics techniques. “Taken together they are capable of using available data more effectively, either by reducing the required volume or by extracting more value from unstructured, diverse data sources,” said Hare.
More Robust Analytics
Small data is an approach that requires less data but still offers useful insights. The approach includes certain time-series analysis techniques or few-shot learning, synthetic data, or self-supervised learning. Wide data enables the analysis and synergy of a variety of small and large, unstructured, and structured data sources.
“Both approaches facilitate more robust analytics and AI, reducing an organization’s dependency on big data and enabling a richer, more complete situational awareness or 360-degree view,” said Hare.
Potential areas where small and wide data can be used are demand forecasting in retail, real-time behavioral and emotional intelligence in customer service applied to hyper-personalization, and customer experience improvement.
Other areas include physical security or fraud detection and adaptive autonomous systems, such as robots, which constantly learn by the analysis of correlations in time and space of events in different sensory channels.
A shift to small data
Gartner predicts that 70 percent of organizations will shift their focus from big to small and wide data by 2025, making AI less data hungry. Companies are realizing that learning from big data is expensive and time-consuming, according to the firm. That’s because machine learning requires many examples, many hours of data labeling, and significant effort to train and tune a model.
Using humans to label large volumes of data is not only labor intensive but also prone to errors that impact the accuracy of the results, added Gartner. “Humans do not learn in this way. We learn from just one or two examples. A person needs to see a wine glass and a coffee cup only once to know the difference. Machines need thousands of wine glasses and coffee cups examples to make the distinction. We need to design algorithms that learn in this way to scale the adoption of AI.”
Other organizations do not have enough historical data to for training algorithms. For instance, lean manufacturers operating at six sigma may have only one defect per million parts – too few for machine learning.
For Avaya IT professionals, the lesson is clear. Understand the assumptions and the data used to develop AI communications applications in order to be sure they can deliver the desired improvements.
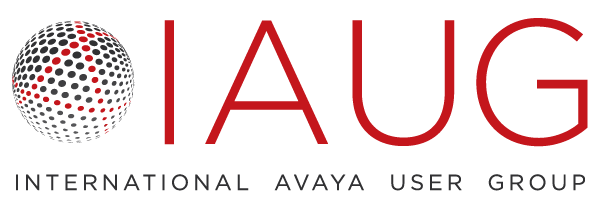