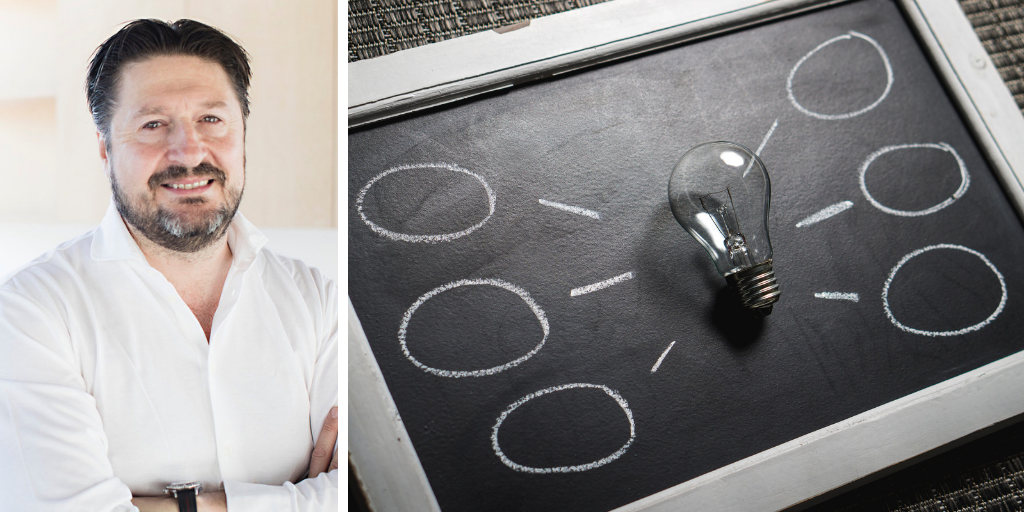
Is your IT team looking at knowledge graphs as a new business intelligence tool? By pulling together relevant information from varied databases, they can provide insights that give savvy organizations a competitive edge in their markets.
What is a knowledge graph?
A knowledge graph is a mathematical framework for gathering and processing a large amount of structured and unstructured data and connecting them in a graph-like model. By mining diverse data sources, the platform creates an efficient algorithm-based representation of the overall picture. Indeed, a knowledge-graph platform uses algorithms to construct relationships even when connections are not evident. It shows the relationships and the nature of their connections and derives time-based inferences suggesting promising areas of intelligence.
The investment “gold” lies in the multiplicity of knowledge connections and trends that the graph can create, allowing IT professionals to be more efficient and creative in discerning relevant tactics and strategies in many areas, such as enterprise data governance and risk management.
How can enterprises use knowledge graphs?
Major enterprises are commonly faced with thousands of isolated “data silos,” and face an information overload challenge. A knowledge graph approach can serve as a centralized framework of integrated knowledge by extracting units of knowledge from those datasets.
Ideally, a knowledge graph continuously “reads” disparate sources, creating an integrated enterprise solution. It can cluster entities, people, events, and ideas into a unified knowledge layer across the institution, while correlating concepts to allow for inference generation and insights.
Knowledge graphs can also be used as semantic search engines sparking new ideas and finding unexpected connections in research and knowledge discovery applications. This applies to connections related directly to the organization and between industries and areas of knowledge.
As a result, a well-constructed knowledge graph, built from a very large quantity of diverse data sets, can be used to make predictions, support decision-making efforts, and identify strategies to find meaningful trends that could take dozens of human analysts hundreds of hours to discern.
How can knowledge graphs benefit the long-term bigger picture?
Knowledge graphs have another potential value to the enterprise: identifying complex risk exposures. Today, there are a constantly increasing number of possible connections (both direct and indirect) affecting a given company, industry, market, or economy. Because knowledge graphs are actual graphs, in the proper mathematical sense, they allow for the application of inference-graph-based techniques.
In that sense, some of the most significant use cases of knowledge graphs relate to reasoning and “inferring relationships” — essentially drawing connections between sometimes disparate events or information that wouldn’t be connected otherwise. This allows for the quantification of risk exposure within a complex contagion framework.
In that sense, some of the most significant use cases of knowledge graphs relate to reasoning and “inferring relationships” — essentially drawing connections between sometimes disparate events or information that wouldn’t be connected otherwise. This allows for the quantification of risk exposure within a complex contagion framework.
Knowledge graphs can be used to quantify company exposure to as AI, robotics, and environmental, social and governance (ESG) themes by processing a variety of sources, such as official filings, government awards, patents, government reports which provide a holistic view of a company’s business, products, services, and intellectual property.
Alternative data – that is, data sets from outside traditional spheres – have become increasingly available with the rise of technologies, but are difficult to gather and process. Shipping logistics data, court filings, patents, clinical/medical reports and the like provide a wealth of information, but the sheer volume makes processing meaningful insights a real challenge.
A comprehensive data graph can draw those inferences, poring over thousands – or tens of thousands – of records to form an alternative data engine that extracts data points from alternative data sources. It can give insight into existing themes, suggest new directions and potentially mitigate risks.
As our ability to collect meaningful data grows, knowledge graphs provide a very practical solution to parse out real knowledge from those diverse pieces.
Ruggero Gramatica is the co-founder and CEO of Yewno.
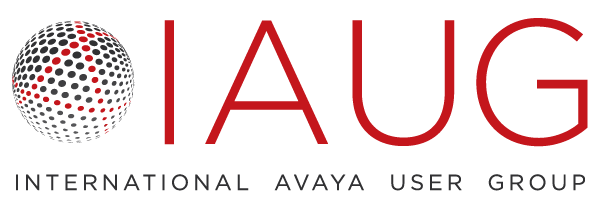
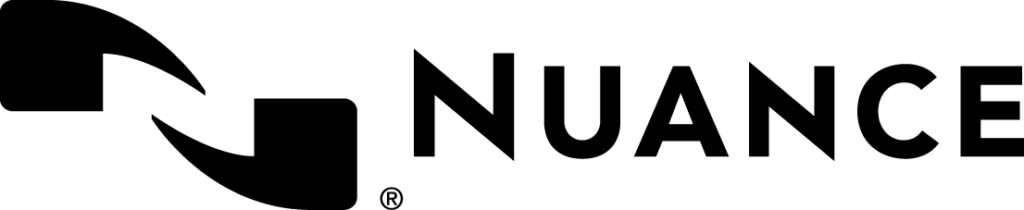